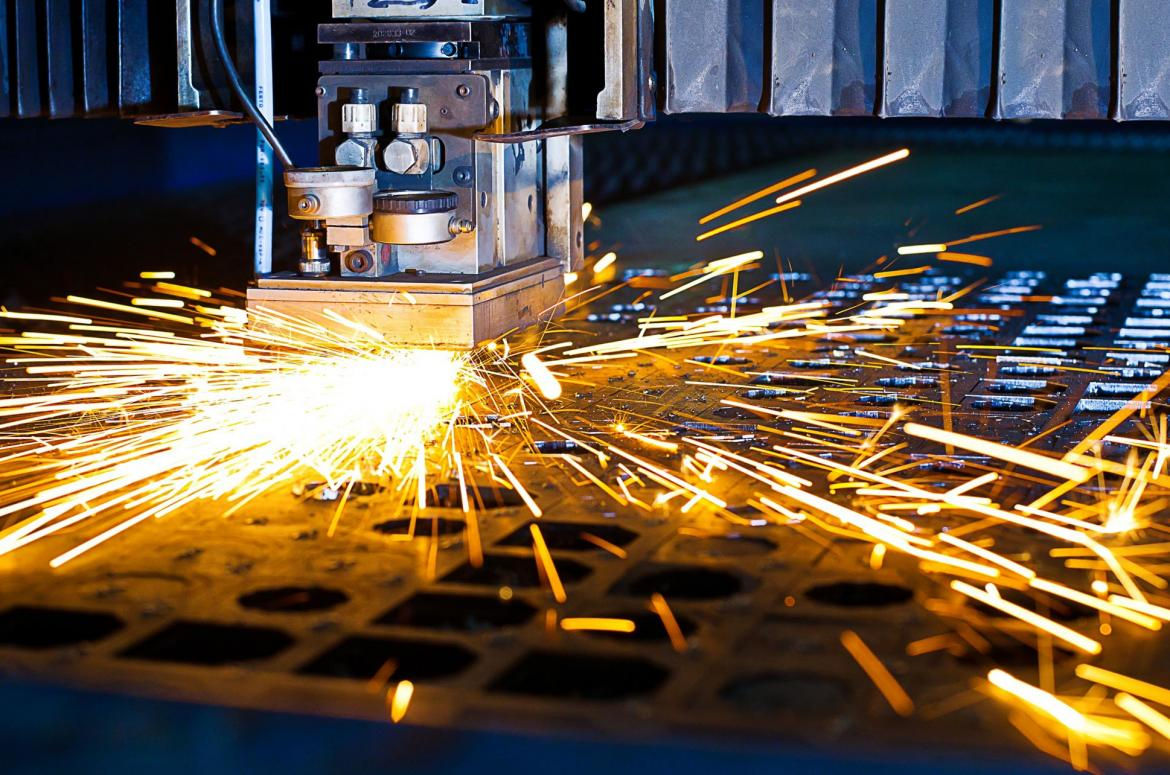
SCHEDULE
__________________________
7:45-8:30AM: Breakfast and Registration
​
8:30-8:45AM: Bruce Lawler, MIT MIMO
Introduction
8:45- 9:25AM: Retsef Levi, MIT ORC
A System Approach to Data Analytics: Designing Intelligent Systems and Processes
This session will provide a system level framework to understand the role new technologies, such as AI, ML, data analytics, automation and sensors can play in the design of intelligent (and innovative) systems and processes and more generally transform organizations and enterprises to become data driven. It will cover basic terminology related to data modeling, as well as descriptive, predictive and prescriptive models. The session will highlight the interaction between data, models and decision processes as key to the process of designing intelligent systems and creating a data-driven organization that leverages an enterprise knowledge system. Another aspect that will be highlighted is the interaction with human and particularly expert operators. Both positive and negative examples will be discussed.
9:25-10:05AM: Ingrid Millan, McKinsey & Company
World Economic Forum Lighthouse Manufacturers - Data Learnings from Davos
44 of the most advanced manufacturing factories in the world are lighting the way as learning beacons. Hear the latest on how advanced manufacturers are applying the technologies of the Fourth Industrial Revolution to drive operational and environmental impact.
​
10:05-10:45AM: Vivek Farias, MIT ORC
How Manufactures can Compete in an Online/Offline World Using Predictive Analytics
A key problem facing retailers and manufacturers is optimizing fulfillment of online orders using inventory that is distributed across multiple supply locations. Predictive analytics provides proprietary insights that allow retailers and manufacturers to optimize inventory across an omnichannel environment.
10:45-11:05AM: Networking Break
Machine Learning in Manufacturing and Operations Use Case Poster Session
Have a chance to view the latest use cases for machine learning in manufacturing and operations. The use cases will cover the types of data used, its source, the analytical approach taken, the KPI improvements and the impact to operations.
11:05-11:25AM: Vice President, Nike
Using Data Acquisition and Predictive Analytics to Anticipate Consumer Needs
Nike greatly accelerates its digital advantage by being insight-driven, data optimized and hyper-focused on consumer behavior. Learn how Nike serves consumers more personally at scale.
11:25-12:00PM: Panel Discussion, Industry Executives and MIT Faculty
Getting The Most Out of Your Data
This panel discussion will address key questions about data: What is the proper management and use of all your data? What is the value of your “dark data”? Should it stay dark? How do I determine which data to validate, store and maintain? How reliable is my data?
​
12:00-1:00PM: Lunch
Break out discussions with Industry Executives and MIT Faculty
-
Data Types and their Treatment - Hierarchal, Spatial, Temporal
-
Sensors and Communications - Industrial Internet of Things/Industry 4.0
-
Data Skills - What Are They? Who Needs Them?
-
Digital Thread: How Can I Create One Among Design, Suppliers, Manufacturing and Service?
1:40-2:10PM: Vijay D'Silva and Ashutosh Dekhne, McKinsey & Company and Bruce Lawler, MIT MIMO
Executive Session
McKinsey & Co. and MIT MIMO will present the results of a comprehensive, first-of-its-kind AI implementation study. The study has identified how manufacturing and operations executives: create the right organization capabilities and structure; focus on solvable problems; and make appropriate budgeting decisions to achieve maximum impact on relevant KPIs, by deploying industry-specific AI applications and solutions.
1:00-1:40PM: Brian Anthony, MIT Sense.nano
New Frontiers in IoT Sensing Technologies
How will the development of new kinds of connected, inexpensive, and widespread sensing devices that harness the power of nanoscale imaging and manufacturing systems impact many of industry’s’ most pressing problems. A deeper look at high speed imaging, laser ultrasound, and product inspection using wide area imaging and 3D ultrasound.
​
2:10-2:30PM: Prakhar Mehrotra, Senior Director of Machine Learning, Walmart
Making sense of Petabytes
Walmart is the world’s biggest retailer with over 20,000 stores in 28 countries and has built the world’ biggest private cloud to process 2.5 petabytes of data every hour. Learn how Walmart has made sense of all of this information and put it to work solving problems with its state-of-the-art analytics hub.
2:30-3:10PM: Aleks Maudry
How Cyber Attacks can Poison Data and Manipulate Machines While They Learn
As cyber security threats to enterprises continue to become more sophisticated, stealthy, and devastating—some of which even weaponize AI technologies - how can you prevent Adversarial attacks on Data? This presentation will show how can bad actors influence machine learning while it’s learning and the different ways can you manipulate machine learning by attacking the data it uses to learn.
3:10-3:25PM: Networking Break
3:25-4:05PM: Tim Kraska, MIT CSAIL
Making “Citizen Data Scientists” Effective
By fundamentally re-thinking the data analytics stack with innovations across : User Interface, Interactive Data Exploration and Automated ML, we can enable a new breed of citizen data scientists. An interactive data science platform will allow users to interact with data and empower them to explore and mine data without requiring programming experience, background in statistics or machine learning expertise.
​
4:05-4:25PM: Leigh Hunnicutt, AMGEN
Using Data, Analytics and Human Factors to Deliver and Analytics
Nowadays, the use of analytics and machine learning has become popular in many areas including manufacturing. Manufacturers have realized that exploiting the data they have available can be key to further helping the success of their business. This has led to a new era where the use of analytics and machine learning in this space has become more prevalent. In this talk, we will discuss how analytics and machine learning can bring an edge in demand forecasting. We will discuss key some challenges ranging from demand forecasting for new products where there is little or no data available to situations where the “right” data is not available.
​
4:25-5:00PM: Georgia Perakis, MIT ORC
Augmenting Demand Forecasting Using Machine Learning Multi-modal Data
Many manufacturers have to deal with a high volume of new items, fast turnover, and frequent design innovation. Short product lifecycles require accurate demand forecasting. This session will discuss using machine learning with many structured and unstructured data types (images, short text descriptions, shipment, purchasing, and sales histories) to improve demand forecasting.
​
5:00-5:05PM: Bruce Lawler
Wrap-up